How to Use Customer Data for Machine Learning
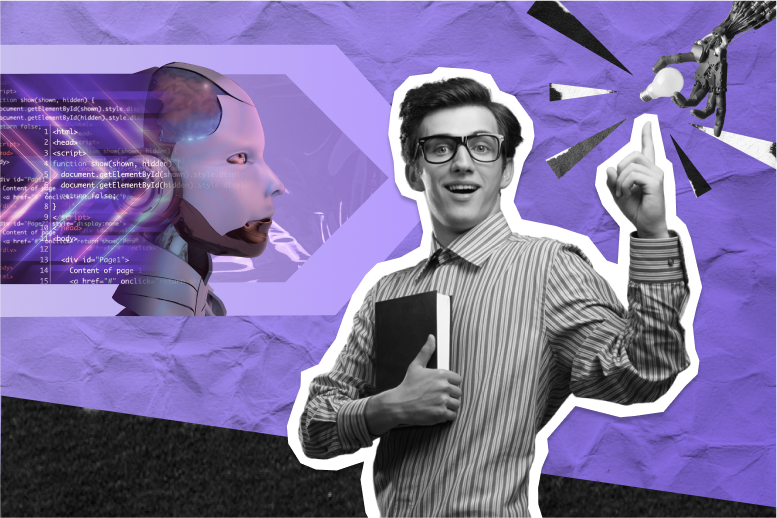
Machine learning (ML) is becoming increasingly important, and those who quickly grasp the functionality of ML will gain an advantage over their competitors. Many companies of varying sizes use purchased technologies to develop marketing strategies, but not all market players accurately assess the suitability of their databases for ML.
Progress does not stand still: companies will be able to derive real benefits from machine learning technology if they learn to collect high-quality source data. The quality of input information directly affects the results of ML.
How to unlock the full potential of machine learning
To ensure that machine learning models function effectively, it is crucial to load them with a large amount of data. The more comprehensive the information you provide, the more productive the results from machine learning will be. Incorrect or contradictory data can lead to inaccurate predictions by the models.
The accuracy of predictions is important for making informed managerial decisions. "Good data" reduces the risk of errors.
Machine learning algorithms rely on the information they are provided to continue learning and improving. Information is also necessary for adapting the model to new conditions and predicting events in current realities.
Terms and definitions in machine learning
Stages of machine learning
Otherwise, this stage can be called 'data cleaning,' which takes up a significant amount of time and effort. If the input information is in the wrong format or lacks proper context, the training will be incomplete, and the model will not produce accurate results.
The route of data
The data journey involves a chain of data transformations, including the collection and processing of input information using machine learning algorithms to enable the model to make predictions and decisions.
Step 1: Data collection
Preparing customer data for machine learning is not an easy task. This question becomes particularly acute when multiple sources of information are involved, both external and internal.
For companies, input information includes user activity on the web, data on purchases made, interactions with customer service, and monitoring of customer activity in mobile applications.
Step 2: Data standardization
Collected data is useless without standardization: it is converted into a format that can be processed by machine learning algorithms, typically CSV or JSON. It is important for the data to be consistent. Duplicate or outdated information is removed, and missing values are compensated for. Data analysts spend a significant amount of time cleaning and harmonizing "dirty" data.
Event specification involves real-time quality checks on incoming data sets. When a new event occurs, the verification is performed. This ensures the cleanliness and alignment of the information for machine learning purposes.
Why do we need data infrastructure?
Customer information is collected and analyzed to improve products according to consumer needs and expectations. In addition to the product itself, channels of promotion and communication with customers are adjusted based on the gathered insights.
A strong information foundation leads businesses to growth and enables the automation of routine tasks. The freed-up time allows employees to focus on developing conceptual solutions. The result is increased workforce efficiency, savings in the marketing budget, and growing company profits.
You might be interested in:
For those who want to implement cross-selling and increase the average order value.
Read moreReactivation is a campaign for inactive subscribers who have not opened your emails, clicked the links and purchased anything for about three to nine months.
Read moreWeb push notifications are contextual, timely, personalized messages featuring a quick-to-the-point alert or call to action. Businesses use these messages to drive engagement back to the website.
Read more